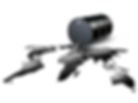
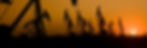
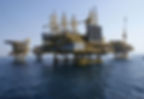
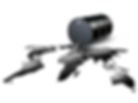
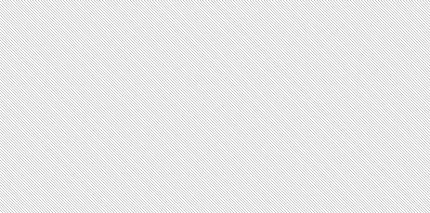
EORServices
Services and Knowledge base

SURFACTANT
The word surfactant stands for the portmanteau of the words ‘Surface’ and ‘Active’. Surfactants are amphiphilic in nature. It has an affinity to a polar medium (water) and a non-polar medium (hydrocarbon). The dual affinity of surfactant molecules result in a mono-layer between two mediums (Schramm. et. al., 2003). This mono-layer causes a decrease in interfacial tension (IFT) and forms a micro-emulsion between oil and water, this micro-emulsion, with low IFT moves with ease thorough the pore space.
The surfactant molecule consists of a hydrophilic head and a hydrophobic tail (Figure 1). The hydrophobic tail (can be either straight or branched) of the surfactant molecule interacts more strongly with the oil molecules while the hydrophilic head has more affinity towards the water molecule (solvation). The solubility of the surfactant molecule depends on the hydrophilic to lipophilic ratio (HLB). This ratio characterizes the tendency of surfactant to solubilize in either oil or water and form water in oil or oil in water emulsions respectively. For instance, higher HLB results in the surfactant molecule being more soluble in oil system and forms water in oil emulsion (Paul Willhite. et al., 1998). Many such surfactant molecules combine together and form micelles. The oil molecules form the interior of the micelle while the exterior or the hydrophilic head of the micelle clings to the water molecules (Figure 2).
The hydrophilic head of the surfactant molecule is a characteristic parameter in defining the types of surfactant, classified as anionic, cationic, non-ionic and zwitterionic (Schramm. et. al., 2003).
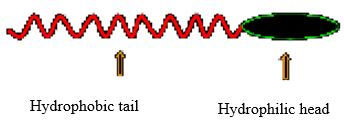
Figure 1 Structure of a Surfactant molecule (Paul Willhite. et al. 1998)
Figure 1 above shows a surfactant molecule with its hydrophilic head and hydrophobic tail, while figure 2 below shows a micelle structure, once surfactant molecules unite. The micelles attribute toward forming micro-emulsions with low IFT values.
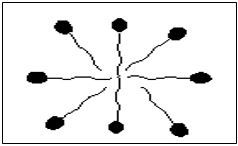
Figure 2 Structure of a surfactant micelle (Schramm. et al. 2003)
-
Types of Surfactants
Surfactants are classified on the basis of the ionic charge of the hydrophillic head of the surfactant as follows:
-
Anionic: As the name suggests anionic surfactants have a negative head group. These negatively charged surfactants help in lowering the IFT and can be manufactured economically. Their biggest advantage lies in their resistant nature to retention which can be attributed to the negative charge of the head group. As the head group is negatively charged, these surfactants repel against the negatively charged interstitial clay. Due to such advantageous properties, anionic surfactants are widely used in EOR techniques. Internal Olefin Sulfonate (IOS), an anionic surfactant, shows good tenacity against high temperature. Such surfactants can be used in reservoirs having high temperature since the stability of the surfactant will remain intact. A vast amount of research has been carried out on IOS surfactants as potential tools in surfactant flooding. Blending of various anionic surfactants to arrive at the best surfactant slug is an idea which has come forward in the 21st century. Levitt et al. studied a blend of IOS and propoxy sulfate and found promising laboratory results.
-
Non-ionic: These surfactants have a head group which has no ionic charge, hence the name non-ionic surfactants. These surfactants are generally used as co-surfactants, albeit after the chromatographic separation effects between the surfactants and the co-surfactants are studied. Common examples include alcohol, ester, ethers, etc.
-
Cationic: These are positively charged surfactants. These surfactants are occasionally used for EOR as they are adsorbed at the surface of interstitial clay due to the negative charge of the clay minerals and the positive charge of the
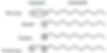
Figure 3 Types of Surfactants (Schramm et al. 2000)
surfactant molecule, this causes attraction between the two which results in loss of expensive surfactant from adsorption. The lower permeability reservoirs might add to their retention by phase trapping.
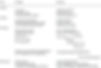
Figure 4 Examples of different types of Surfactants (Schramm et al. 2003)
-
Zwitterionic (Amphoteric): These surfactants have a negative as well as a positive group head as shown in figure 5. Zwitterionic surfactants are known for their robust structure, high tolerance to salinity and temperature (Alhasan Fuseni et al. 2013). Needless to say, these surfactants are used in harsh reservoirs and have immense potential for EOR in future. A healthy amount of research is already underway (Zhou Xianmin et al. 2012, Bataweel et al. 2012).

Figure 5 Diagram showing structure of Gemini surfactant molecule (Bo Gao et al.2013)
Besides these four general surfactant types, there also exist a few other less used surfactants like Viscoelastic surfactants (VES) and Gemini surfactants. Gemini surfactants are described as dimeric surfactants as the surfactant molecules have two head groups and two tails per surfactant molecule, which are linked by spacer group (Shramm. et al. 2000). Developed in late 1980s and early 1990s, these surfactants can either be Cationic, Anionic, Non-ionic or Zwitterionic Gemini depending on the hydrophilic head of the surfactant molecule. The great advantage of Gemini surfactants over single-tail, single-head surfactants is that, they have low Critical Micelle Concentration (about an order of magnitude) (CMC) and can be used in low permeability reservoirs. They also exhibit high surface activity, better stability, low IFT at CMC and high hard-water tolerance. Figure 2.5 shows the basic structure of a Gemini surfactant molecule.
MICROEMULSION AND CMC
The term micro-emulsion was first used by Schluman et.al in 1977. These micro-emulsions form colloidal solution. The use of the word micro-emulsion was in debate after it was coined, Shinoda’s and Kunieda’s work stands a case in point. According to Healy and Reed, a micro-emulsion is defined as a stable, translucent micellar solution of oil, water that may contain electrolytes and one or more aphiphilic compounds (surfactant, alcohol) (Healy and Reed, 1974).
Micro-emulsions contain micelles that solubilize the immiscible phase with the solvent in the micro-emulsion solution. These micelles are called as swollen micelles. With the right amount of concentration of micro-emulsions, a significant amount of oil can be solubilized.
At low concentration of a surfactant in a solution, the surfactant molecules are dispersed randomly. These random surfactant molecules are called as monomers. However, as the concentration of the surfactant molecules increases, they start aggregating together to make the insoluble phase soluble. The coalesced surfactant molecules form a sphere, like a droplet of a liquid substance, which are called as micelles. These micelles later form a micro-emulsion.
The micelles only start forming after a certain value of a surfactant concentration. This value is called the Critical Micelle Concentration of a given surfactant. Once, the concentration has reached CMC and micelles are formed, the surfactant monomers stop getting dispersed in the solution but start getting added into the micelles. This is an important phenomenon when surfactants are used in EOR techniques. This can be explained by stating that after reaching CMC the surfactant injection should be stopped as the added surfactant will only aggregate with micelles and not contribute to further IFT reduction. Adding more surfactant to the solution after achieving CMC will cause its wastage and increase the expenditure of the EOR project.
When a surfactant is added to the immiscible phases of water and oil, they form micelles which convert the immiscible phases into a single solution. The single solution formed can either be water in oil type or oil in water type. This helps in increasing the microscopic sweep efficiency. Microscopic sweep efficiency as the name suggests increases the mobility of the oil bank formed by the surfactant micelles on the scale of pore spaces. This simply means that the solution of water and oil moves with more ease in the pore spaces of the reservoir.

Figure 6 Micelles Left: oil-in water, Right: water-in oil (Paul & Willhite., 1998)
Figure 6 shows 2 different types of micro-emulsions a surfactant can form with the oil-water system, while figure 7 exhibits the formation of micelle at critical micelle concentration of surfactants.

Figure 7 Formation of Micelles from monomers (Paul & Willhite., 1998)

Figure 8 Graphical representation of relationship between CMC and Micelles
PHASE BEHAVIOR
Phase behavior studies of surfactants slugs to evaluate the robustness and IFT reduction capacity were carried out in the era of 1980s and 1990s (Puig J. E. et al. 1979, Hall A. C. 1980). Micro-emulsion systems between oil, water and surfactant can be designed which have ultralow IFT’s (order of magnitude 10-3 dynes/cm). This is one of the mechanisms of surfactant flooding.
Phase behavior studies are tedious as the micro-emulsion systems are sensitive to the structure and concentration of surfactant slug (which includes surfactant, co-surfactant (usually an alcohol or another surfactant), oil and brine water), temperature and pressure. Phase behavior studies evaluate regions where solubilization caused by micelles is maximum and the micro-emulsion is least affected by the above mentioned parameters. In literature, there are not any universally accepted mathematical equations which can be used to evaluate the phase behavior studies of surfactant. This results in laboratory evaluation of the micro-emulsions, where micro-emulsion structures are studied experimentally. The results obtained from the experiments are produced in the form of graphs which can be used in different computer softwares (for instance UTCHEM) to create mathematical model.
Healy and Reed in 1974 studied the phase behavior of surfactants with the help of ternary diagrams. The concept of ternary diagram was introduced on the basis of the principle that the micro-emulsion at least consist of three components namely, oil, water and surfactant. The most ideal system was considered to have these three phases after the equilibrium between the three components was achieved. The system with an ideal amount of all the three phases was a stabilized one. There exist three types of scenarios for a system. The type of system will depend on how the phase behavior changes take place while the three phases try to achieve equilibrium. These three types are Winsor type I, Winsor type II and Winsor type III. Winsor type I forms a lower phase equilibrium region, which means the lower phase micro-emulsion attains equilibrium with the oil above it. This type is also referred as Winsor type II-, where II means that two phases exist in the system. In this system the solubility of the surfactant is more towards the brine region (lower phase) as there is an electro-static force acting continuously between the surfactant ions and the uneven distribution of water dipole (O- H+). The water dipole moment results in solubilization of surfactant in the brine region due to which IFT of the system remains high. Only a small amount of oil is seen in the solubilized region. Winsor type II system exists when surfactants solubilize the upper phase region of the system with the lower excessive brine phase region. This type also has only two phases co-existing together. The lower region phase is purely aqueous with brine water and the upper solubilized phase with excessive oil. This type will not reduce the IFT adequately for the oil to be produced. Winsor type III shows a co-existence of water, oil and surfactant in the micro-emulsion. When the solution reaches type III state equilibrium is established between the three phases mentioned above and results in the formation of an oil bank in the middle region occupied by the micro-emulsion. Winsor type III is considered the most productive as it reduces the IFT to the least value when compared to the other 2 types. The varying salinity conditions from the optimal salinity requirement cause variations in the type III system.
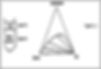
Figure 9 Winsor type I system (salager et al., 1979)
Figures 9, 10 and 11 show the winsor types behavior I, II and III respectively. Winsor type III behavior is considered the most ideal of the micro-emulsions a surfactant can form with an oil-water system.

Figure 10 Winsor type II system (salager et al., 1979)

Figure 11 Winsor type III system (salager et al., 1979)
Healy and Reed and Healy et.al. studied the effect of salinity on the phase behavior of a micro-emulsion. It was found that the stability of three phase micro-emulsion which has the lowest value of IFT exists only for a selected range of salinity and the mid-point of this range was the value called as optimum salinity value (S*). Optimum salinity gives the right amount of density to the micro-emulsion to solubilize required amount of water and oil in the middle region. However, if the salinity is more than the optimal salinity, the micro-emulsion region witnesses change from the required type III system and transform’s into type I or type II with increase or decrease in salinity respectively (Figure 12)

Figure 12 Effect of changing salinity on type III system (Paul & Willhite 1998)
Figure 12 as explained shows the effect of salinity on the Winsor type micro-emulsion formed by surfactants and figure 13 shows the actual test done to check the effect of salinity on the ability of surfactant to form micro-emulsions.

Figure 13 Micro-emulsion type changes with increasing salinity to the right (Ted Davis et al., 1980)
-
Phase Behavior Observation
Phase behavior testing is an important part of the screening process of surfactants. Phase behavior testing is carried out for a surfactant at a particular temperature, hydrocarbon and surfactant concentration.
Generally, a small quantity (usually 2ml) of sample of oil-water-surfactant mixture is pipetted into a long pipette (usually 5 ml) and then placed in an oven with a specific temperature set to match the reservoir temperature. After the temperature is reached the pipettes are inverted several times. The solutions in the pipettes are later studied to observe the phase behavior.
The solution is studied to observe the changes in the micro-emulsion region. It is also studied to observe the changes in Winsor types the micro-emulsion system goes through. The salinity is kept on increasing in small intervals to study the effect of salinity and parameters like Optimal salinity (S*) and Solubilization ratio (σ*) are measured through graphs. For a given system of oil-surfactant-water, solubilization ratio is the volume oil and water being solubilized by a unit amount of surfactant (Paul & Willhite 1998). Figure 2.14 from a DOE report of surfactant evaluation shows the graphical method of obtaining the value of S* and σ*.

Figure 14 Solubilization and Optimal salinity graphs (Hirasaki et al., DOE report 2004)
SURFACTANT RETENTION
Surfactant retention occurs due to adsorption, phase trapping or precipitation. Adsorption of surfactants on the solid-substrate of the rock (especially in carbonate reservoirs) is one of the important factors in determining the success of a surfactant flooding process. A great amount of surfactant is lost due to adsorption which results in increasing the cost of the project and rendering the flooding impractical. In a typical project half or more of the total project expenditure is attributed to the surfactant cost (K. O. Meyers et al. 1981). Therefore, surfactant adsorption studies are imperative in a surfactant chemical EOR method project.
Surfactant retention by precipitation is generally caused by the temperature effects on the surfactants. Precipitation occurs due to the dissolution of surfactant elements into salts (Kleppe J and Skjeveland S.M 1992). Therefore it is essential to design a surfactant which is robust enough against dissolution in salt. Here, the pH of the surfactant plays a major impact on the resultant adsorption. Hardness which is generally considered as the concentration of divalent ions (Ca2+, Mg2+) also is an important parameter controlling the amount of surfactant adsorption. Diffusion of surfactants on pores of a rock (phase trapping) also causes surfactant retention (Cuong T. Q Dang et al., 2011).
Adsorption mainly occurs due to the charged head groups of solid surface and the charged hydrophilic head of the surfactant (Figure 15). Generally, a single monomer of surfactant is adsorbed on the rock surface rather than micelles being adsorbed as a whole. (Somasundaram et al., 2000). Adsorption of surfactants depends on the surfactant type, concentration, molecular weight, pH, salinity and importantly on reservoir heterogeneity.
Langmuir adsorption isotherms (or simply adsorption isotherms) are used right from 1980’s (EOR boom period) till today (J. F. Scamehorn et al., 1980, Cuong T. Q Dang et al., 2011). The isotherm curve is a plot of Surfactant adsorption Vs Surfactant concentration.
These curves generally show a steep rise in the surfactant adsorption, occurring when the surfactant is in the Winsor type I and type II region. Once, the surfactant concentration hits the CMC value, the adsorption remains fairly constant as shown by most of the curves. This region is called a plateau and is of considerable importance. The CMC value is usually 100 times more than the surfactant concentration at the start of injection and the aim of adsorption isotherms is to note the adsorption value at the CMC since this is the highest adsorption value the surfactant slug attains. Hence, these factors suggest that the shape of the curve below CMC value has little impact on the total surfactant adsorption (Cuong T. Q Dang et al., 2011).

Figure 15 Surface adsorption on the rock surface (Cuong T. Q Dang et al., 2011)
The aforementioned adsorption curve is derived from the experiments carried out in the laboratories, where a surfactant under test is exposed to the reservoir rock (either crushed or in the form of a core). Static adsorption method comprises of crushed rock pieces being placed in a small tubes along with surfactants. The temperature is varied to notice its role in surfactant adsorption. The liquid/solid system is then well mixed by centrifugation and random hand agitation.
Dynamic adsorption method consists of rock cores being flooded with surfactant slug to study the adsorption. The surfactant concentration is gradually increased until CMC is reached and the curve shows a remarkable plateau.
SURFACTANT MECHANISMS
Surfactants reduce the IFT between oil and water by emulsifying them. It also results in wettability changes from oil wet to water wet used in surfactant imbibition EOR in fractured carbonate reservoirs. Reduction in IFT results in an enhanced microscopic displacement efficiency. To have a desired volumetric sweep efficiency (mobility control), polymers are added after injecting surfactants. This method is called as Surfactant/Polymer EOR.
-
Interfacial Tension
Interfacial tension or IFT is the contractile tendency at the liquid-liquid interface (for instance oil/water) when the two immisicible liquids are in contact. It is the force per unit length which is required against the contractile forces to create more surface area (Paul & Willhite, 1998). In reservoir rocks the oil and brine IFT is between 20-30 dynes/cm as shown in figure 16. Due to such high IFT the residual oil saturation and remaining oil saturation of the reservoir is higher. Surface active agents like surfactants can be used to reduce the IFT by creating more surface area at the junction of the two liquids. This will increase the microscopic displacement efficiency of the reservoir and reduce the residual oil saturation.

Figure 16 IFT between Oil, gas and brine phases (Yildiray Cinar et al., 2005)
Capillary number is the ratio of viscous forces over capillary forces (Saffman and Taylor 1958). In literature, there are many other definitions of capillary number. Mathematically it is denoted by Nc.
Nc = vµ/σ
Where:
Nc is capillary number
V is the effective velocity
µ is the viscosity of the displacing fluid
σ is the IFT between oil and water
CDC is an ideal method to co-relate residual oil saturation to the physical properties (like capillary forces, IFT) on a microscopic sclae (pore scale) (Bashiri A. et al., 2011). Taber in 1979 showed that, the inversely proportion relationship between capillary number and IFT can be used as a method to decrease the residual oil saturation. CDC which show that when capillary number is increased the residual oil saturation decreases. He also suggested that the capillary number of the reservoir system after waterflooding is somwhere close to 10-7. The low value of capillary number after waterflooding can be increases if surfactants are used in the water system (LTWF) which will reduce the IFT of the system and in turn increase the capillary number. CDC are plots of ROS Vs Nc as shown in the figure 17.

Figure 17 Capillary Desaturation Curve (CDC) (Lake, 1989)
The viscous forces, directly proportional to the capillary number depend on the permeability of the reservoir, the applied pressure drop and the viscosity of the displacing fluids while the capillary forces depend on the IFT of oil and water, wettability conditions and pores geometry (M. Delshad et al., 1986). The aforementioned parameters on which the viscous and capillary forces depend suggest that increasing the viscous forces to increase the capillary number is not feasible (for instance, there is always a danger of damaging or fracturing the formation if the viscosity of the displacing fluid is more than the fracture pressure of the formation. IFT is measured with the help of spinning drop tensiometer (V.J. Kremesec et al., 1988).
SURFACTANT FLOODING AND TYPES
Surfactant flooding Surfactant flooding is broadly classified into two namely, Micellar-polymer (Surfactant polymer flooding) and Alkaline-Surfactant-Polymer flooding (ASP) (Paul & Willhite, 1998). Any deviation from the aforementioned methods is subtle, for instance, surfactants can be added to water while waterflooding to decrease the capillary forces between the injected water and oil resulting in better recovery efficiency. This method is called as low tension water-flooding (Gogarty W.B., 1978). Second subtle change from the main method is by adding foam instead of polymer for mobility control purposes.
Despite of few subtleties, the above mentioned two methods remain the imperative methods which have been piloted and also implied on a large scale in a few reservoirs. These surfactant flooding methods and their application is described extensively in the following sections.
-
Micellar/ Polymer Flooding.
A micellar/polymer flooding operation employs a micellar solution consisting of oil, water, surfactant and small amounts of other chemicals like, co-surfactants (alcohol, other surfactants) polymer. These chemicals together make the micellar/ polymer flooding slug. This method is also called as Micro emulsion flooding and Surfactant-polymer flooding (Sara T. et al., 1992).
A Micro-emulsion of oil/surfactant/water exists in the form of drops of the size of microns. Hence, this method improves the microscopic efficiency of the reservoir. Micellar/polymer method was first used and patented for Marathon oil co. by Gogarty and Tosch known as Maraflood. The injection profile of the method consists of injecting a pre-flush (to achieve the desired salinity environment), followed by micellar slug (surfactant, co-surfactant, electrolyte), which is followed by polymer solution along with drive water. Figure 18 below shows the injection profile of the flooding method.

Figure 18 Micellar/ Polymer flooding injection profile (Sara T. et al., 1992)
The method establishes low IFT between oil and water and forms an oil bank which is eventually produced.
-
Alkaline Surfactant Polymer (ASP) Flooding
Alkaline surfactant flooding method comprises of injecting alkaline (NA2CO3, KOH) followed by surfactant and polymer. The main objectives of the method are to reduce the loss of surfactants by retention, changing the wettability and reducing the IFT of the fluids. Alkaline chemicals reduce the surfactant retention, increase the pH value and also react with the acidic content of hydrocarbons (Naphthenic acids) to generate more surfactants in the reservoir. The intensity with which the alkaline substance reacts with the acids depends on the acid number of the hydrocarbons. The Polymer noticeably is used to improve the mobility conditions. They provide the necessary volumetric efficiency to the system. Extensive research is been carried out in ASP methods at the turn of 21st century. Field applications are noticeable in the USA and on a large scale in China.
HA + OH- Ã A- + H2O
Where, A- is the soap component formed
HA- Naphthenic acid component
SURFACTANT EVALUATION
Screening criteria of any EOR method is considered as the first step towards evaluating a successful EOR project. Virtually since three decades, EOR techniques have been evaluated as new technologies emerge in the EOR industry. Due to this emergence in technology it is critical to always keep the screening criteria updated. Screening criteria is extremely important as the first step to any EOR evaluation as a massive amount of money is invested to apply an EOR method. Hence, to avoid the risk of a failure every EOR method is carefully evaluated for a particular set of oil and reservoir properties (Hite 2004). The objective of a screening criteria operation is to impeccably estimate a specific range on reservoir and oil properties in which various EOR methods are applicable (Paul & Willhite, 1998).
Surfactant evaluation is carried out to test the efficacy of a single surfactant or a mixture of various surfactants. Surfactant structure, concentration and suitable values of oil properties like oil viscosity, brine salinity, reservoir temperature, formation mineralogy are various parameters for which the surfactant is evaluated (Hirasaki et al., 2004). The surfactant type is hampered and limited by the reservoir temperature and salinity (Paul and Willhite, 1998).
According to Hirasaki et al., surfactant evaluation must take into account the chemical/physical conditions in reservoirs, economic factors, commercial availability of surfactants and type of surfactant method applied. It is important to evaluate the surfactant to check its interaction with the type of reservoir rock. For instance, the physical properties of the sandstone rock vary when compared with carbonate rocks. Hence, different surfactant methods are evaluated for these rocks. Sandstone rocks are generally not fractured while carbonate reservoirs mostly have a fracture network. Surfactant imbibition method is evaluated for carbonate rocks. It is also important to know the aqueous phase chemistry of the reservoir and its interaction with surfactant. In order to study the aforementioned relationship, surfactants are characterized by the optimal salinity (S*) (at which the surfactant are the most stable) for different hydrocarbon specimen. Solubilization parameter estimates the level of IFT reached at the optimal conditions. In surfactant evaluation, surfactants are monitored for formation of viscous gel or liquid crystals which change the micro-emulsion composition and hamper favorable IFT values and eventually the recovery factor.
Over the past 20 years, many researchers have developed and published technical screening criteria for different EOR techniques. Table 2.3 shows the screening criteria for surfactant flooding published by different researchers. The EOR screening studies presented by Brasher and Kuuskraa (1978) had a dataset of 200 EOR pilot projects in the USA. They analyzed the data from both a technical and an economic perspective. Carcoana (1982) presented screening criteria for some EOR techniques; these criteria were based on the pivotal knowledge of reservoir properties and the results obtained from commercial applications of EOR techniques in Romanian oil fields. Taber (1983, 1997) proposed screening criteria based on field data and oil recovery mechanisms for commonly applied EOR techniques. This study considered the 1996 Worldwide EOR Survey to summarize the criteria. Taber’s criteria that are relevant surfactant flooding include that the maximum oil viscosity should be less than 35 cP, and reservoir permeability should be greater than 10 md. He presented these screening criteria both graphically and in tables. Goodlett et al. (1986) presented screening criteria based on a summary of previously published screening criteria for chemical, gas injection, thermal, and microbial EOR techniques. Al Bahar et al. (2004) illustrated criteria for each EOR technique based on the literature and his own experience. He utilized software to evaluate the suitability of these criteria for EOR processes at 81 reservoirs in Kuwait. In addition, a novel improved hydrocarbon recovery (IHR) screening methodology has been developed to identify the appropriate process for any number of reservoirs. (Table belowshow only the criteria for Surfactant-Polymer flooding). Subsequently, a range for field and laboratory data was established considering the reservoir and fluid parameters. The work also included salinity and hardness parameters for which are imperative for chemical flooding. Also, the laboratory dataset included parameters such as IFT, surfactant adsorption and surfactant concentration which are important in defining the success of surfactant-polymer flooding.
Screening guide for surfactant-polymer flooding
A dataset consisting of Surfactant-Polymer field projects (Micellar-Polymer, Micro-emulsion projects) from worldwide EOR survey biennially published in the Oil & Gas Journal was established. The authenticity of the data collected from the Oil & Gas Journal was verified from SPE publications on the respective projects. Eventually, the dataset acquired consisted of 42 field projects. Additionally, the study comprised of dataset from laboratory work carried out in surfactant-polymer flooding. The laboratory dataset consisted of 200 experiments which were acquired from SPE literature (onepetro) dedicated toward these experiments. The following work explains the data analysis and range established from the acquired field and laboratory dataset.
FIELD PROJECTS
-
Data Cleaning
The quality of data collected plays a major role in establishing a genuine screening criteria result for any EOR process. The collected data might contain problems that can affect the quality of dataset, in particular, duplicate projects, missing data, inconsistent data and special cases.
The problem of duplicate and inconsistent information in the dataset was solved by referring to the SPE work published on the field projects. All the published work referred therefore, provided an authentic dataset of field projects free from inconsistent and duplicate information. The special cases were however, analyzed by studying the relationship between box-plots and cross-plots for the different parameters.
Finally, the distribution of Surfactant-Polymer flooding projects applied in different countries is shown in figure 19. It can be conferred from the figure that approximately 79% of the field projects were applied in USA.

Figure 19 World surfactant-polymer flooding projects (From Oil & Gas Journal EOR surveys)
The remaining 9 field projects were applied in Germany, France, Indonesia, England, Russia and China with 3 field projects in China, 2 in France, while the remaining countries conducted 1 field project each.
-
Missing Data
Some fields in the dataset were missing one or more pieces of information. The missing data included °API gravity, Oil Saturation (start and end), salinity and hardness. Table below shows details of the missing information which were ignored in the analysis and the aforementioned parameters which were missing in the field projects in the dataset. It provides with the percentage value of each missing parameters along with the number of available and unavailable data for the same parameters. Brine hardness had the most amount of missing data with the percentage of 69.00%.
Data unavailable for each parameter in the dataset
-
Data Problem Detection
The dataset was analyzed for special cases and a few inconsistent data. To interpret these problems, basic diagrams viz. box plots and cross plots were used.
Box-Plot: In descriptive statistics box-plot is a quick and efficient way to analyze the data. It helps in visually summarizing the data and spot the special cases. The special cases for a given parameter are the values which lie segregated from the majority of the data-points. The box-plot also gives the range of the values which fall between the minimum and maximum limit. It is divided into 5 parts. The characteristic features of a box-plot can be explained as follows.
-
The lowest value (minimum)
-
The highest value (maximum)
-
The mean value (Average data value)
-
The first quartile (25th percentile)
-
The second quartile (50th percentile)
-
The third quartile (75th percentile)

Figure 20 Schematic of a box-plot
Figure 20 is a depiction of a box-plot with its characteristic features. The figure explains the concept of special cases, which are values larger and smaller than the upper and the lower limit of the data respectively. The upper limit of the data is calculated as 1.5 the interquartile range plus the 75th quartile, while the lower limit as 1.5 times the interquartile range minus the 25th quartile. The interquartile range is the difference between the 75th quartile and the 25th quartile. The mean is the dot at the center of the plot.
Cross-Plot: A Cross-plot is an X-Y plot which is used to interpret a relationship between two different parameters. It is a plot with scattered points which follow a specific trend. Hence, a trend-line is achieved which shows the trend of the scattered data of the two parameters. A cross-plot combines well with the box-plots of the two parameters and helps in better understanding of the data-points which lie separated from the majority of the points.
Figure 21 shows a cross-plot of temperature vs. depth to the left and temperature box-plot to the right. Generally, the temperature of a formation depends on the depth with temperature increasing with depth as the geo-thermal gradient increases with depth.
It can be inferred from the box-plot of temperature (figure 21, right) that all the temperature values of the fields lie below the upper limit of 214.75 which is denoted by the solid black line. These values did not show inconsistency with depth as shown in the cross-plot of temperature vs. depth. The upper limit in the temperature box-plot exceeded
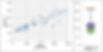
Figure 21 Cross-plot of reservoir temperature vs. depth (left) and temperature box-plot (right)

Figure 22 Box-plot of Depth
The maximum value of the temperature due to the mathematical formula of [Q3+1.5*(interquartile)] by which it is calculated. The field project applied in Sloss area of Nebraska was considered as a unique project for its high temperature value. The other 2 temperature values of 191°F and 185°F close to the maximum value were recorded for the reservoirs in Arkansas. The field names were Wesgum and Lewisville respectively. Figure 22 depicts the box-plot of the reservoir depths for 40 field projects with the maximum value of 7500 feet and a minimum value of 475 feet. The maximum value was recorded for the field of East Coalinga Extension in California, USA while the minimum value was for St. Johnson field of Illinois, USA.

Figure 23 Cross-plot of porosity vs. permeability (left) and permeability box-plot (right)
Figure 23 above shows a cross-plot relationship of porosity vs. permeability to the left and the permeability box-plot for permeability values in the dataset. Values of 5 field projects exceeded the upper limit value of 1050.3md. These field projects were applied in Bell Creek, USA (2 field projects) and three field projects in China (2 in Shengli and 1 in Bohai Bay) with permeability values of 1050, 1218 for Bell Creek field and 1320, 2000 and 1300 md for oil fields in China respectively. Three field projects of viz. Chateaurenard, (France), Westblock 4 in Germany and Handil oilfield in Indonesia had reservoir permeability values of 1000md, approximately 50md less than the upper limit. However, these values were still higher than the permeability values for the majority of field projects. All these projects were conducted in unconsolidated sand formations which are highly permeable. These were the special cases which were not included in defining the final range of permeability for SP flooding as all the other projects were carried out in sandstone reservoirs. Figure 24 shows a porosity box-plot for forty one porosity values. The upper limit and the lower limit of the plot are depicted, with the upper limit above the maximum value whisker and lower limit below the minimum value whisker.

Figure 24 Reservoir porosity box-plot.
The porosity box-plot showed a wide spread between 11 and 34%. The upper and lower limit of the plot was higher than the maximum and lower than the minimum values respectively. This was attributed to the formula with the help of which these values are calculated.

Figure 25 Cross-plot of oil viscosity vs. oil gravity (left) and oil gravity box-plot (right)
Figure 25 shows a cross-plot between oil viscosity and oil gravity and oil gravity box-plot to the right. Three oil viscosity values lie away from the trend shown by the majority of the values. These values of 40 cP, 25 cP and 30 cP were of the field projects applied in Chateaurenard, France and Willmington, USA. Bohai Bay off-shore project was conducted for oil viscosity of 30 cP. Chateaurenard reservoir had a high viscosity oil as the oil had no dissolved solution gas which made the oil thick. The oil also contained a large amount of paraffinic content. Similarly, the oil in Willmington reservoir was stripped of solution gas. The waterflooding history of the reservoir also attributed to the increase in viscosity of the oil. Since, low temperature water was injected in the reservoir during waterflooding, the temperature of the reservoir dropped considerably thereby, increasing the viscosity of the oil.
Similarly, it can also be inferred from figure 26, which is box-plot of oil viscosity, that two field projects with reservoir oil viscosity of 25, 30, 40, 45 and 50 cP. These values were higher than the upper limit of 24.0785 cP with a maximum value of 50 cP observed in the Shengli oilfield (Northwest block) in China.
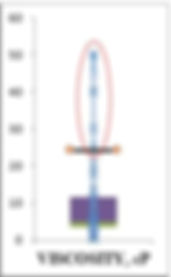
Figure 26 Oil Viscosity box-plot
Figure 27 and figure 28 show box-plots for Brine salinity and brine hardness respectively. Brine Salinity values showed a wide range from 400ppm to a maximum of 160,000ppm. Field projects in Loudon, Wichita and Manvel reservoir of USA had high salinity values than the majority of the values in the dataset. A minimum value of 400ppm was of the reservoir brine of Chateaurenard, France oilfield. The upper limit was calculated to be 175,350ppm which was
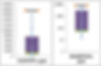
Figure 27 and 28 Box-plot of brine salinity (left) and brine hardness box-plot (right)
Figure 28 is a box-plot of brine hardness vs. brine salinity and a box-plot of brine hardness to the right. As seen from the box-plot 8 values from the 13 brine hardness values lie in the range of 13-231 ppm. Five hardness values were observed to be above 1000ppm. Three of these values are of the field projects applied at Salem, North-Burbank and Manvel all in the USA, with the brine hardness values of 3800, 6530 and 2400 ppm respectively. Brine hardness value of 3000ppm and 4000ppm were observed in the fields of Eldorado and Loudon located in Kansas and Illinois states of the United States. Brine hardness is the concentration of divalent ions (Ca2+ and Mg2+ in the brine). Brine hardness is an important parameter and has to be studied as high concentration of divalent ions in the brine results in the precipitation of the surfactant slug and affects its stability. This eventually, increases the cost of the entire Surfactant-Polymer flooding project.
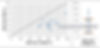
Figure 29 Cross-plot of oil saturation (start) vs. oil saturation (end) (left) and Oil saturation (start) box-plot (right)
Figure 29 shows a cross-plot of oil saturation (start) vs oil saturation (end) and a box-plot of oil saturation start values in the dataset. Also, figure 30 shows a box-plot of oil saturation end values. On the X-axis we have the oil saturation start value and on the Y-axis, oil saturation end values were plotted. The cross-plot has a line which passes from the minimum value of 0 to the maximum value of 60. Any point over this line would mean that the oil saturation end value is more than the oil saturation value at the start of Surfactant-polymer project, which cannot be possible. It can be inferred from the cross-plot that no project showed such inconsistency. However, the Surfactant-polymer project applied at the Botthamsall field in the United Kingdom shows a high final oil saturation value of 50% (also seen in the box-plot- Figure 30). This was attributed to the low permeability of the reservoir (6md) which caused high retention of the chemical slug. The box-plot of Oil saturation start shows 2 field projects in Arkansas- USA with values of 87% and 96%. These high values suggest that the water-flooding recovery from these reservoirs was low.

Figure 30 Box-plot of Oil saturation (End)
-
Methods to Display Data
After analyzing the data for special cases and consistency for different parameters, the dataset values were displayed with the help of histograms and box-plots.
Histograms: Histograms are used to display dataset values graphically and show data points in specified ranges. They also show the frequency of the data on the Y-axis and the parameters being measured on the X-axis.
Figure 31 shows a histogram of reservoir temperature of the dataset. It shows that the Surfactant-polymer chemical flooding is implemented in different ranges of reservoir temperature from 65-200°F. It also shows that the minimum value of reservoir temperature at which surfactant-polymer flooding method was implemented at 65°F, while highest was at 200°F. The highest peak was observed between 65-80°F. Approximately, 71% of the temperature values lied between 70-120°F.

Figure 31 Reservoir temperature range of the dataset
Figure 32 below shows a histogram of reservoir depth for 41 surfactant-polymer chemical flooding projects. The figure shows that the peak for reservoir depth is between 1000-2000 feet. The majority of the values fall between the reservoir’s depths of 500-2000 ft, with more than 50% of the values falling in this range. Only 1 project lies between the highest value range of 7000 and 7500 ft.

Figure 32 Reservoir depth range of the dataset

Figure 33 Porosity range of the dataset
Figure 33 shows a histogram of reservoir porosity of the dataset for 40 field projects. The highest frequency porosity lies between 17 to 23%. Approximately 52% of the porosity values lie in this range. The maximum porosity value of 34% is shown between 31-34%.
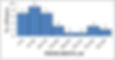
Figure 34 Reservoir permeability range of the dataset
Figure 34 shows a histogram of reservoir permeability of sandstone formations. The permeability range is across 35 field projects. 7 permeability values of reading above 1000md were excluded as special cases. These fields had high values as they consisted of unconsolidated formation. Majority of the permeability values lie in the range of 6-150md. Approximately 71% of the permeability values lie between 10-150md. The minimum value of 6md was of the reservoir in Bothamsall field of the United Kingdom.

Figure 35 Oil viscosity range of the dataset
Figure 35 shows a histogram of oil viscosity of the dataset across 42 field projects. The figure is shows high frequency to the left. The highest peak is for the range of 3-6 cP. Approximately, 72% of the viscosity values are in the 0-12 cP range. Few oil viscosity values ranged from 21-50 cP, with the maximum value of 50 cP. The values of 45 and 50 cP were observed for the field projects conducted in China.
Figure 36 shows a histogram of oil gravity °API of the dataset across 39 field projects. It can be inferred from the histogram that most of the values lie in the range of 28-42°API suggesting that Surfactant-Polymer flooding projects are generally applied in reservoirs containing light oil. The distribution, as can be seen is skewed to the left. Approximately, 72% of the oil gravity values lie between the 28-41° API range.
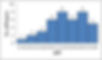
Figure 36 Oil gravity range of the dataset
Figure 37 shows the histogram for oil saturation (start). The dataset for oil saturation (start) comprise of 35 field projects. The highest peak in the distribution lies between 30% and 35%. The diagram is skewed to the right. Also, 66% of the data values fall between 30 and 40%. This is usually the residual oil saturation at the end of water-flooding.
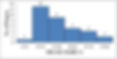
Figure 37 Oil saturation (start) range of the dataset
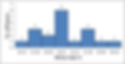
Figure 38 Oil saturation (end) distribution of the dataset
Figure 38 shows the oil saturation (end) distribution of the dataset across 16 data points ranging from 10 to 28%. The highest oil saturation (end) frequency occurs between 19 and 22%. Approximately, 69% of the oil saturation (end) values fall between 13 and 22% range.
Box-plot: The box-plots as described earlier were used to detect the special cases and inconsistent data in the dataset. However, they were also used to display the ranges and summarize the dataset for each parameter. Figure 39 shows the box-plots for different parameters for surfactant-polymer chemical flooding process. Data value ranges were provided for each parameter (minimum and maximum value) after omitting the data analyses. The minimum and maximum value range was illustrated by the distance between the opposite end of the whiskers. Additionally, the box-plots also show information such as mean and median of each parameter.
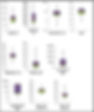
Figure 39 Left- Right-Box plots of Reservoir temperature, Depth, Oil viscosity, Oil gravity, Reservoir porosity & permeability, Brine Salinity & Hardness, Oil saturation start & end
Figure 3.21 shows box-plots of all the important parameters of the field projects. The box-plots show the mean and median values of all the parameters along with the minimum and maximum values denoted by the whiskers.
LABORATORY DATA
Most of the screening criteria work which has been published so far consists of field projects and pilot projects for various EOR processes. However, continuous laboratory research is been carried out to improve EOR processes. A good amount of research is also carried out in Surfactant-polymer chemical flooding process.
A dataset of laboratory work was established comprising of experiments for Surfactant-polymer flooding method. The dataset source comprised of information obtained from various publications in the SPE literature of onepetro. The dataset includes different experiments published to study properties and efficiency of surfactant-polymer flooding. All the injection experiments were carried out in various cores to study the efficiency of the flood for oil recovery.

Figure 40 Laboratory surfactant-polymer flooding experiments from 1970-2013
Figure 40 shows the distribution of laboratory work on surfactant-polymer flooding carried out over the years. It shows the number of experiments which were conducted from 1970-2013. The turn of 20th century saw the most number of experiments carried out to check the efficiency of SP flooding. Sixty experiments in our dataset were executed in the years 2000 to 2013. Also, 99 experiments took place from 1970 to 1990, which is EOR boom period.
Figure 41 shows the different surfactants which were used in the experiments to establish a surfactant slug. Including the 4 basic surfactants such as Anionic, Non-ionic, Cationic, and Zwitterionic surfactants, there emerged a class of Bio-surfactants, which are generated through microbes and bacteria. The pie-chart (figure 3.22), illustrates the percentage and number of experiments conducted in each category. Zwitterionic dataset was the smallest as this class of surfactants was only recently proposed for research.

Figure 41 Distribution of types of Surfactants used in laboratory dataset
Conventional, anionic surfactants were used in most of the experiments, resulting in the biggest dataset. Over 79% of 200 experiments used anionic surfactants to study their effect on oil recovery.
Figure 42 depicts the type of polymers used in the dataset. It shows the percentage and number of 4 different types of polymer viz. Hydrolyzed polyacrylamide, xanthan gum, bio-polymer and associating polymer used in the experiments. HPAM was the most common polymer used; while only a few experiments were conducted using a new emerging class of Associating polymer.

Figure 42 Types of Cores used in the Laboratory dataset
Sandstone cores, sandpacks and carbonate cores were the 3 major types of cores used in the tests. More than 80% of the tests were conducted in the sandstone cores including Berea, Bentheimer, Briarhill and Reservoir cores. Few tests (14%) were conducted in sandpacks, while even fewer were conducted in non-fractured carbonate cores.
Data Analysis
Data analysis included representing the data with the help of box-plots, histograms and cross plots.
Cross plots were used to analyze the related parameters for example, core porosity vs. core permeability, the Oil viscosity vs. Oil gravity, Oil recovery (% of residual oil saturation after water-flooding) vs. Surfactant slug concentration and oil viscosity.
In descriptive statistics, a box-plot is a statistical way to graphically describe a group of numerical data by their quartiles. The box-plot used in this study divides the set of data into 6 parts as follows.
-
The lowest value (minimum)
-
The highest value (maximum)
-
The mean value (Average data value)
-
The first quartile (25th percentile)
-
The second quartile (50th percentile)
-
The third quartile (75th percentile)
Figure 43 shows a schematic of a box-plot used to display the data. Histograms, as previously used to depict the range of different reservoir and oil parameters for field projects, were also used to observe the range of different laboratory parameters.

Figure 43 Schematic of a box-plot
Figure 44 shows a cross-plot of oil viscosity vs. oil gravity of the crude oil used in different tests. One test, as is evident by the diagram showed oil viscosity of 376 cP and gravity of 16.5°F. A test using such heavy oil was conducted to study the efficiency of the surfactant-polymer flooding process in heavy oil reservoirs of Canada.

Figure 44 Cross plot of Oil viscosity vs. oil gravity
It shows a very few data-points as some experiments used crude oil with same oil viscosity and oil gravity. Also, many experiments only mentioned the oil viscosity and not the oil gravity °API.

Figure 45 Oil viscosity histogram (left) and oil viscosity box-plot (right)
Figure 45 depicts the histogram and box-plot of oil viscosity values used in the experiments. The lowest oil viscosity value was of 0.5cP while the highest oil viscosity value was of 550cP, which is denoted by the >32 bar in the histogram. It can be inferred from such high oil viscosities that the surfactant-polymer flooding is being tested for recovery efficiency of heavy crude oil, to increase the application range of the method.

Figure 46 Oil Gravity histogram (left) and oil gravity box-plot (right)
Figure 46 shows the range of oil gravity °API with the help of histogram and the box-plot. The minimum value was 14.5° API and further the low values observed were between the values of 14.5 to 20°API. Such low values were observed for the experiments in which heavy crude oil with high viscosity were used. The new proposed class of zwitterionic (amphoteric) surfactants was tested for recovery in heavy oil reservoirs. A few experiments were conducted for a crude oil with viscosity of 75cP. An Alkoxy Carboxylate surfactant with large hydrophobic tail was used to check its stability in the presence of high viscosity oil. Oil recovery results for these experiments showed promising results.
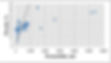
Figure 47 Cross-plot of core permeability vs. core porosity
Figure 47 shows a cross-plot of core permeability vs. core porosity. The data-points show a consistent relationship between the porosity and permeability values. However, five peremeability values lie on the higher side, over 1000md. These high permeability values were observed for sandpacks. Sandpacks are the loosely packed grains of sand which are generally used to observe the behaviour of the chemical slug in the formation and its interaction when it comes in contact with the crude oil. The minimum value observed in the cross-plot was of 106 md of reservoir core.

Figure 48 Core porosity histogram (above) and core porosity box-plot (below)
Figure 48 represents the range of core porosity with the help of a histogram and a boxplot. As we study the diagram, it can be inferred that the highest peak of core porosities was observed in the range of 18 to 22. Approximately, 76 percent of the porosity values lie between 14-26%. The lowest value of porosity observed was of 6.47%. This value was the porosity value measured for a reservoir core. The porosity box-plot shows a spread of porosity values between 6.47 to 41%, with the mean at 23.9%.
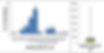
Figure 49 permeability histogram (above) and permeability box-plot (below)
Figure 49 shows the distribution of permeability values for the different cores used in the tests. It can be concluded from the histogram and permeability box-plot that the permeability values are spread over a wide range from 9.8md to over 5000md. Majority of the values in the dataset lie between 70 and 800. However, the permeability values vary significantly from one core to other core, for instance, one of the reservoir core permeability was 2668md, while most of the reservoir cores had permeability values of less than 1000md. Also, the high permeability values (greater than 1000md) are observed for sandpacks and few Bentheimer cores.
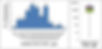
Figure 50 Surfactant slug concentration histogram (left) and box-plot (right)
Figure 50 gives the range for surfactant slug concentration used in the tests in our dataset. Majority of the values lie in the range of 5000 to 40,000 parts per million (ppm). Approximately 77% of the 175 surfactant concentration values lie in the aforementioned range. It can also be inferred that a few values are as low as 2.8ppm. Concentrations of bio-surfactants used in a few experiments had a range of 2.8ppm to 41ppm. The box-plot of surfactant slug concentration shows a mean of 20,355.6ppm. High concentration of surfactant slug (over 50,000ppm) in the subset was observed for tests which used surfactant blend of petroleum sulfonate and non-ionic surfactant. The concentration measured was a combination of these surfactants.

Figure 51 Surfactant slug size histogram (left) and box-plot (right)
Figure 51 depicts the range of surfactant slug size in terms of pore volume injected. The surfactant slug size of the subset ranged from 0.03 to 3. As can be inferred from the plots, majority of the values lie between 0.041 and 0.9. The surfactant slug size over 1PV were used in bio-surfactant tests.

Figure 52 Polymer drive concentration histogram (left) and box-plot right
Figure 52 illustrates the range of polymer drive concentration (parts per million) with the help of a histogram and box plot. Majority of the tests were conducted with polymer concentration of 800 to 2000 ppm. 74 of the 121 values in the subset lie in this range. Few values in the dataset were high in the range of 4000-5000ppm. These were bio-polymer concentrations.
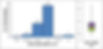
Figure 53 Polymer drive slug size histogram (left) and box-plot (right)
Figure 53 gives the range of polymer drive slug size in terms of total pore volume injected. The minimum value observed in the dataset was 0.35 PV while the maximum value observed was 2.8 PV. Majority of the values in this subset were in the range of 0.5PV to 1.5 PV. The maximum slug size of 2.8 PV was observed for a test conducted in reservoir sandstone core to achieve a favourable mobility ratio.
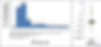
Figure 54 IFT between oil-water-surfactant system histogram (left) and box-plot (right)
Figure 54 shows the interfacial tension (IFT) values between oil-surfactant-water system. It can be inferred that the IFT values in the dataset had a wide spread from 0.0001 dynes/cm to 4.2 dynes/cm. The higher IFT values were observed for the oil-surfactant-water system in which bio-surfactants were used. Also, as can be interpreted from the IFT box-plot, the mean of the subset 0.274 dynes/cm because of these high values ranging from 1 dynes/cm to 4.2 dynes/cm.
Figure 55 shows the range of dynamic adsorption of anioinic surfactants on sandstone adsorbent. The adsorption was measured in terms of milligrams of surfactant adsorbed per gram of adsorbent. Majority of the adsorption values ranged from 0.1mg/g to 0.6mg/g. Few of the surfactants had high surfactant adsorption, ranging from 1 mg/g to 4.2 mg/g. These were observed for reservoir sandstone cores, where adsorption increases due to reservoir heterogenity.
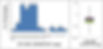
Figure 55 Dynamic adsorption histogram (left) and box-plot (right)

Figure 56 Static adsorption box-plot
Figure 56 shows the range of static adsorption of anionic surfactants on kaolinite adsorbent. It can be inferred from the box-plot that the adsorption ranges from 0.4 mg/g to as high as 66.1 mg/g. When compared with dynamic adsorption, static adsorption has higher values. This can be attributed to kaolinite adsorbent used in the static adsorption experiments. Since clay has higher surface area than sandstone, more surfactant is adsorbed on it.

Figure 57 Brine salinity histogram (right) and box-plot (left)
Figure 57 shows the brine salinity distribution with the help of a histogram and a box-plot. It can be inferred from the graph that the minimum and maximum values for brine salinity was 478ppm and 235,000ppm respectively. Such high brine salinity values were observed for surfactants which were tested for harsh reservoir conditions of salinity and hardness.
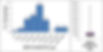
Figure 58 Brine hardness histogram (right) and box plot (left)
Figure 58 illustrates the distribution of brine hardness subset. It can be inferred that the brine hardness values had a wide spread from 130ppm to as high as 40,000ppm. The high hardness values were observed in some tests conducted to check surfactant stability in harsher reservoir conditions conataining hard brines.
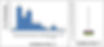
Figure 59 Temperature histogram (left) and box plot (right)
Figure 59 shows the range of temperatures under which the experiments were conducted. The values show a wide range of spread between 20°C to 177°C. It can be inferred from the figure that few experiments were conducted under high temperatures for instance; one test was conducted at a temperature of 177°C (350°F) to test the stability of surfactant (akyl aryl sulfonate) at such high temperature.
Figure 60 illustrates a cross plot of Surfactant slug concentration (parts per million) vs. the tertiary oil recovery of fraction of oil in place after waterflooding for all the tests performed. The oil recovery, as can be concluded varies significantly from core to core. This prominent difference between oil recoveries for the cores can be attributed to the type of cores being used to check the efficiency of the surfactant-polymer flooding. Berea sandstone cores, Briarhill sandstone cores, Bentheimer sandstone cores were used in most of the tests. These standard cores are more homogenous than the reservoir cores.

Figure 60 Cross-plot of surfactant concentration vs. tertiary oil recovery (after waterflooding)
Low residual oil recovery in the reservoir cores was attributed to the heterogeneity of the rock, which affected the stability of the chemical slug which resulted in higher surfactant retention (adsorption) and affected the micro-emulsion phase, which in turn affected the size of the oil bank formed. Surfactant slug concentration injected in the cores also affected the tertiary oil recovery. For example, in tests where low concentration of bio-surfactants was used, the oil recovery was poor. However, when high concentration of surfactants (47,600 ppm) was injected in a core, all of the residual oil was recovered as indicated by the cross-plot above. Figure 61 shows the range of percentage of tertiary oil recovery after waterflooding. The values of the subset had a wide spread between 19.5% to 100% tertiary oil recovery. Most of the low oil recoveries were observed for reservoir sandstone cores, while higher tertiary oil recoveries were measured for more homogenous Berea sandstones, Briarhill sandstones.
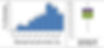
Figure 61 Tertiary oil recovery histogram (right) and box-plot (left)
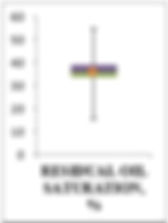
Figure 62 Residual oil saturation box-plot
SUMMARIZING THE FIELD DATASET
Table below gives the range for surfactant-polymer flooding on the basis of statistical analysis for a cleaned dataset. The summary includes all the parameters which eventually decide success or failure of a surfactant-polymer flooding project. The statistical parameters used to define the range were mean, median, standard deviation, minimum and maximum values.
The ranges for the parameters was eventually compared with the previous work and the differences were noted.
-
The concluded summary of oil gravity had a range from 17°API to 45°API. Brasear (1978), Carcaoana (1982) and Goodlett (1986) suggested a value greater than 25°API, while Taber (1997) suggested a value to be greater than 20°API. Aldasani and Bai gave a range of 22°API to 39°API, with and average value of 34.8°API.
-
Oil viscosity in the study has a range of 0.32cP to 50cP, while authors like Caracoana (1982), Peter H. (2984) and Goodlett (1986) suggested a value less than 30cP. On the other hand, Taber (1997) suggested a value of less than 35cP for oil viscosity. Aldasani and Bai concluded a range of 15.6 to 3 cP and a mean of 9.3cP.
-
Temperature range in the study was from 65°F to 200°F. Most of the authors suggested the value to be less than 200°F (Table 2.3), while Caracoana (1982) and Al-Bahar (2004) suggested a value less than 180°F and 158°F respectively. The temperature range differed from previous work conducted by Aldasani and Bai, as they concluded a range of 122-155°F with a mean of 138.5°F
-
Depth range was concluded to be between 475 ft to 7500ft which differed from the values of less than 7000ft and 9000ft suggested by Caracoana (1982) and Goodlett (1986) respectively.
-
Porosity value ranged from 11 to 34% in the study. However, Brashear (1978), Caracoana (1982) and Goodlett (1986) suggested a value of greater than 20%.
-
Permeability range was concluded to be between 6md and 500md. This range differed from the value proposed by most of the authors. Brashear (1978), Caracoana (1982), Peter H. (1984), Goodlett (1986), Taber (1997) and Al-Bahar (2004) suggested a value greater than 20md, 35md, 40md, 40md, 40md and 50md respectively.